All books / Book
Spectral Clustering and Biclustering: Learning Large Graphs and Contingency Tables
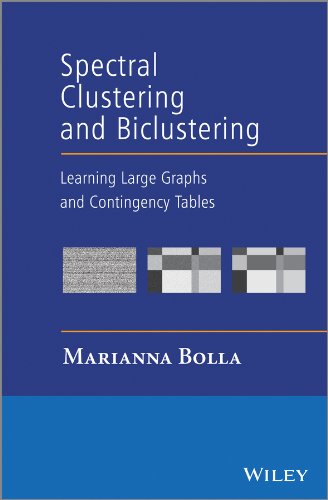
Full title: | Spectral Clustering and Biclustering: Learning Large Graphs and Contingency Tables |
---|---|
ISBN: | 9781118344927 |
ISBN 10: | 1118344928 |
Authors: | Bolla, Marianna |
Publisher: | Wiley |
Edition: | 1 |
Num. pages: | 292 |
Binding: | Hardcover |
Language: | en |
Published on: | 2013 |
Read the reviews and/or buy it on Amazon.com
Synopsis
Offering A Timely And Novel Treatment Of Spectral Clustering And Biclustering Of Networks, This Book Bridges The Gap Between Graph Theory And Statistics By Giving Answers To The Demanding Questions That Arise When Statisticians Are Confronted With Large Weighted Graphs Or Rectangular Arrays. The Author Presents A Wide Range Of Classical And Modern Statistical Methods Adapted To Weighted Graphs And Contingency Tables. In Addition, Practical Examples From Social And Biological Networks Are Included, And A Teacher's Guide Is Provided On A Supporting Website-- Provides A Timely, Novel And Unified Treatment Of Many Important Problems Surrounding The Spectral And Classification Properties Of Networks-- Machine Generated Contents Note: Dedication Preface Acknowledgements List Of Abbreviations Introduction 1 Multivariate Analysis Techniques For Representing Graphs And Contingency Tables 1.1 Quadratic Placement Problems For Weighted Graphs And Hypergraphs 1.1.1 Representation Of Edge-weighted Graphs 1.1.2 Representation Of Hypergraphs 1.1.3 Examples For Spectra And Representation Of Simple Graphs 1.2 Svd Of Contingency Tables And Correspondence Matrices 1.3 Normalized Laplacian And Modularity Spectra 1.4 Representation Of Joint Distributions 1.4.1 General Setup 1.4.2 Integral Operators Between L2 Spaces 1.4.3 When The Kernel Is The Joint Distribution Itself 1.4.4 Maximal Correlation And Optimal Representations 1.5 Treating Nonlinearities Via Reproducing Kernel Hilbert Spaces 1.5.1 Notion Of The Reproducing Kernel 1.5.2 Rkhs Corresponding To A Kernel 1.5.3 Two Examples Of An Rkhs 1.5.4 Kernel -- Based On A Sample --^ And The Empirical Feature Map References 2 Multiway Cut Problems 2.1 Estimating Multiway Cuts Via Spectral Relaxation 2.1.1 Maximum, Minimum, And Ratio Cuts Of Edge-weighted Graphs 2.1.2 Multiway Cuts Of Hypergraphs 2.2 Normalized Cuts 2.3 The Isoperimetric Number And Sparse Cuts 2.4 The Newman-girvan Modularity 2.4.1 Maximizing The Balanced Newman-girvan Modularity 2.4.2 Maximizing The Normalized Newman-girvan Modularity 2.4.3 Anti-community Structure And Some Examples 2.5 Normalized Bicuts Of Contingency Tables References 3 Large Networks,^ Perturbation Of Block Structures 3.1 Symmetric Block Structures Burdened With Random Noise 3.1.1 General Blown-up Structures 3.1.2 Blown-up Multipartite Structures 3.1.3 Weak Links Between Disjoint Components 3.1.4 Recognizing The Structure 3.1.5 Random Power Law Graphs And The Extended Planted Partition Model 3.2 Noisy Contingency Tables 3.2.1 Singular Values Of A Noisy Contingency Table 3.2.2 Clustering The Rows And Columns Via Singular Vector Pairs 3.2.3 Perturbation Results For Correspondence Matrices 3.2.4 Finding The Blown-up Skeleton 3.3 Regular Cluster Pairs 3.3.1 Normalized Modularity And Volume Regularity Of Edgeweighted Graphs 3.3.2 Correspondence Matrices And Volume Regularity Of Contingency Tables 3.3.3 Directed Graphs References 4 Testable Graph And Contingency Table Parameters 4.1 Convergent Graph Sequences 4.2 Testability Of Weighted Graph Parameters 4.3 Testability Of Minimum Balanced Multiway Cuts 4.4 Balanced Cuts And Fuzzy Clustering 4.5 Noisy Graph Sequences 4.6^ Convergence Of The Spectra And Spectral Subspaces 4.7 Convergence Of Contingency Tables References 5 Statistical Learning Of Networks 5.1 Parameter Estimation In Random Graph Models 5.1.1 Emalgorithmfor Estimating The Parameters Of The Block Model 5.1.2 Parameter Estimation In The _ And _ Models 5.2 Nonparametric Methods For Clustering Networks 5.2.1 Spectral Clustering Of Graphs And Biclustering Of Contingency Tables 5.2.2 Clustering Of Hypergraphs 5.3 Supervised Learning References A Linear Algebra And Some Functional Analysis A.1 Metric, Normed Vector, And Euclidean Spaces A.2 Hilbert Spaces A.3 Matrices References B Random Vectors And Matrices B.1 Random Vectors B.2 Random Matrices References C Multivariate Statistical Methods C.1 Principal Component Analysis C.2 Canonical Correlation Analysis C.3 Correspondence Analysis C.4 Multivariate Regression And Analysis Of Variance C.5 The K-means Clustering C.6 Multidimensional Scaling C.7 Discriminant Analysis References Index . Marianna Bolla. Includes Bibliographical References And Index. Mode Of Access: World Wide Web.